Yuting Qiu, James Meng and Baihua Li*
Volume5-Issue1
Dates: Received: 2023-12-30 | Accepted: 2024-01-23 | Published: 2024-01-24
Pages: 055-063
Abstract
Falls are a pervasive problem facing elderly populations, associated with significant morbidity and mortality. Prompt recognition of falls, especially in elderly people with cognitive or physical impairments who cannot raise the alarm themselves, is a challenge. To this end, wearable sensors can be used to detect fall behaviour, including smartwatches and wristbands. These devices are limited by their intrusiveness, require user compliance and have issues around endurance and comfort, reducing their effectiveness in elderly populations. They can also only target patients already recognised as falls risks, and cannot apply to non-identified patients. Leveraging state of the art AI deep learning, we introduce two types of automated fall detection techniques using visual information from cameras: 1) self-supervised autoencoder, distinguishing falls from normal behaviour as an anomaly detection problem, 2) supervised human posture-based fall activity recognition. Five models are trained and evaluated based on two publicly available video datasets, composed of activities of daily living and simulated falls in an office-like environment. To test the models for real-world fall detection, we developed two new datasets, including videos of real falls in elderly people, and more complex backgrounds and scenarios. The experimental results show autoencoder detectors are able to predict falls directly from images where the background is pre-learned. While the pose-based approach uses foreground body pose only for AI learning, better targeting complex scenarios and backgrounds. Video-based methods could be a potential for low-cost and non-invasive falls detection, increasing safety in care environments, while also helping elderly people retain independence in their own homes.
FullText HTML
FullText PDF
DOI: 10.37871/jbres1872
Certificate of Publication
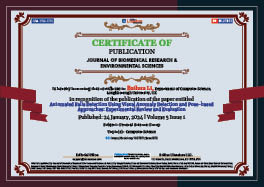
Copyright
© 2024 Qiu Y, et al. Distributed under Creative Commons CC-BY 4.0
How to cite this article
Qiu Y, Meng J, Li B. Automated Falls Detection Using Visual Anomaly Detection and Pose-based Approaches: Experimental Review and Evaluation. J Biomed Res Environ Sci. 2024 Jan 24; 5(1): 055-063. doi: 10.37871/jbres1872, Article ID: JBRES1872, Available at: https://www.jelsciences.com/articles/jbres1872.pdf
Subject area(s)
References
- Moncada LVV, Mire LG. Preventing Falls in Older Persons. Am Fam Physician. 2017 Aug 15;96(4):240-247. PMID: 28925664.
- Montero-Odasso M, van der Velde N, Martin FC, Petrovic M, Tan MP, Ryg J, Aguilar-Navarro S, Alexander NB, Becker C, Blain H, Bourke R, Cameron ID, Camicioli R, Clemson L, Close J, Delbaere K, Duan L, Duque G, Dyer SM, Freiberger E, Ganz DA, Gómez F, Hausdorff JM, Hogan DB, Hunter SMW, Jauregui JR, Kamkar N, Kenny RA, Lamb SE, Latham NK, Lipsitz LA, Liu-Ambrose T, Logan P, Lord SR, Mallet L, Marsh D, Milisen K, Moctezuma-Gallegos R, Morris ME, Nieuwboer A, Perracini MR, Pieruccini-Faria F, Pighills A, Said C, Sejdic E, Sherrington C, Skelton DA, Dsouza S, Speechley M, Stark S, Todd C, Troen BR, van der Cammen T, Verghese J, Vlaeyen E, Watt JA, Masud T; Task Force on Global Guidelines for Falls in Older Adults. World guidelines for falls prevention and management for older adults: a global initiative. Age Ageing. 2022 Sep 2;51(9):afac205. doi: 10.1093/ageing/afac205. Erratum in: Age Ageing. 2023 Sep 1;52(9): Erratum in: Age Ageing. 2023 Oct 2;52(10): PMID: 36178003; PMCID: PMC9523684.
- Kristoffersson A, Lindén M. A Systematic Review of Wearable Sensors for Monitoring Physical Activity. Sensors (Basel). 2022 Jan 12;22(2):573. doi: 10.3390/s22020573. PMID: 35062531; PMCID: PMC8778538.
- Zhang S, Li Y, Zhang S, Shahabi F, Xia S, Deng Y, Alshurafa N. Deep Learning in Human Activity Recognition with Wearable Sensors: A Review on Advances. Sensors (Basel). 2022 Feb 14;22(4):1476. doi: 10.3390/s22041476. PMID: 35214377; PMCID: PMC8879042.
- Jobanputra C, Bavishi J, Doshi N. Human activity recognition: A survey. Procedia Comput Sci. 2019;155:698-703. doi: 10.1016/j.procs.2019.08.100.
- Gupta N, Gupta SK, Pathak RK, Jain V, Rashidi P, Suri JS. Human activity recognition in artificial intelligence framework: a narrative review. Artif Intell Rev. 2022;55(6):4755-4808. doi: 10.1007/s10462-021-10116-x. Epub 2022 Jan 18. PMID: 35068651; PMCID: PMC8763438.
- Bank D, Koenigstein N, Giryes R. Autoencoders. In: Machine learning for data science handbook: data mining and knowledge discovery handbook. 2023;353-374.
- Zheng C, Wu W, Chen C, Yang T, Zhu S, Shen J, Kehtarnavaz N, Shah M. Deep learning-based human pose estimation: A survey. ACM Comput Surv. 2023;56(1):1-37. doi: 10.48550/arXiv.2012.13392.
- Kwolek B, Kepski M. Human fall detection on embedded platform using depth maps and wireless accelerometer. Comput Methods Programs Biomed. 2014 Dec;117(3):489-501. doi: 10.1016/j.cmpb.2014.09.005. Epub 2014 Oct 2. PMID: 25308505.
- Auvinet E, Rougier C, Meunier J, St-Arnaud A, Rousseau J. Multiple cameras fall dataset. DIRO-Université de Montréal, Tech. Rep. 2010;8:1350(24).
- Sabokrou M, Fathy M, Hoseini M. Video anomaly detection and localization based on the sparsity and reconstruction error of auto‐encoder. Electron Lett. 2016;52(13):1122-1124. doi: 10.1049/el.2016.0440.
- Hasan M, Choi J, Neumann J, Roy-Chowdhury AK, Davis LS. Learning temporal regularity in video sequences. IEEE Conf. Computer Vision and Pattern Recognition. 2016;733-742.
- doi: 10.48550/arXiv.1604.04574.
- Nogas J, Khan SS, Mihailidis A. Fall detection from thermal camera using convolutional LSTM autoencoder. Workshop on Aging, Rehabilitation and Independent Assisted Living, IJCAI Workshop. 2018.
- Nogas J, Khan SS, Mihailidis A. DeepFall: Non-Invasive Fall Detection with Deep Spatio-Temporal Convolutional Autoencoders. J Healthc Inform Res. 2019 Dec 18;4(1):50-70. doi: 10.1007/s41666-019-00061-4. PMID: 35415435; PMCID: PMC8982799.
- Redmon J, Farhadi A. Yolov3: An incremental improvement. arXiv preprint arXiv:1804.02767. 2018 Apr 8. doi: 10.48550/arXiv.1804.02767.
- Fang HS, Xie S, Tai YW, Lu C. Rmpe: Regional multi-person pose estimation. IEEE Int. Conf. Computer Vision. 2017;2334-2343.
- Yan S, Xiong Y, Lin D. Spatial temporal graph convolutional networks for skeleton-based action recognition. AAAI Conf. Artificial Intelligence. 2018;32(1). doi: 10.1609/aaai.v32i1.12328.