Przemyslaw Waliszewski*
Volume4-Issue9
Dates: Received: 2023-09-02 | Accepted: 2023-09-14 | Published: 2023-09-15
Pages: 1290-1307
Abstract
Similarity between patterns of cellular self-organization in primary tumor and in its normal counterpart underlies the concept of biological tumor aggressiveness. That subjective parameter is supposed to correlate with both dynamics of local tumor growth and the risk of progression. Subjective evaluation during the microscopic examination is inaccurate. The co-application of fractal geometry and algebraic topology offers a novel perspective. A set of prostate carcinomas comprising all patterns of self-organization was classified according to complexity and entropy to investigate how complexity relates to topology of the patterns. Prostate carcinomas evolve from order to disorder along the increasing entropy as many physical dynamic complex systems do. Carcinomas can be classified into the classes of complexity equivalence according to both the values of the global fractal dimensions D0 or D1 and the Shannon entropy H. Topological measures, such as the bottleneck distance or the Wasserstein distance reveal different topology in very low- and low-aggressive prostate carcinomas (class C1, C2, C3) in comparison with high-aggressive carcinomas (class C6, C7). This is contingent on the appearance of infiltrating cancer cells. While the persistent homology entropy Hph remains similar in all classes, the persistent barcode entropy Hpb is significantly decreased in class C1 and increased in class C7 in comparison with the other ones. Patterns of self-organization can now be defined according to changes in intercellular connectivity by the set of complexity, diversity, homogeneity, and topology measures.
FullText HTML
FullText PDF
DOI: 10.37871/jbres1798
Certificate of Publication
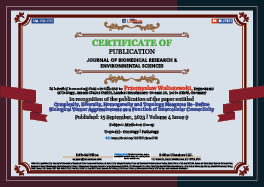
Copyright
© 2023 Waliszewski P. Distributed under Creative Commons CC-BY 4.0
How to cite this article
Waliszewski P. Complexity, Diversity, Homogeneity and Topology Measures Re-Defi ne Biological Tumor Aggressiveness as a Function of Intercellular Connectivity. 2023 Sep 15; 4(9): 1290-1307. doi: 10.37871/jbres1798, Article ID: JBRES1798, Available at: https://www.jelsciences.com/articles/jbres1798.pdf
Subject area(s)
References
- Kweldam CF, van Leenders GJ, van der Kwast T. Grading of prostate cancer: a work in progress. Histopathology. 2019 Jan;74(1):146-160. doi: 10.1111/his.13767. PMID: 30565302; PMCID: PMC7380027.
- Paner GP, Gandhi J, Choy B, Amin MB. Essential Updates in Grading, Morphotyping, Reporting, and Staging of Prostate Carcinoma for General Surgical Pathologists. Arch Pathol Lab Med. 2019 May;143(5):550-564. doi: 10.5858/arpa.2018-0334-RA. Epub 2019 Mar 13. PMID: 30865487.
- Waliszewski P, Wagenlehner F, Gattenlöhner S, Weidner W. On the relationship between tumor structure and complexity of the spatial distribution of cancer cell nuclei: a fractal geometrical model of prostate carcinoma. Prostate. 2015 Mar 1;75(4):399-414. doi: 10.1002/pros.22926. PMID: 25545623.
- Waliszewski P. The Quantitative Criteria Based on the Fractal Dimensions, Entropy, and Lacunarity for the Spatial Distribution of Cancer Cell Nuclei Enable Identification of Low or High Aggressive Prostate Carcinomas. Front Physiol. 2016 Feb 11;7:34. doi: 10.3389/fphys.2016.00034. PMID: 26903883; PMCID: PMC4749702.
- Montironi R, Cheng L, Lopez-Beltran A, Scarpelli M, Mazzucchelli R, Mikuz G, Kirkali Z, Montorsi F. Original Gleason system versus 2005 ISUP modified Gleason system: the importance of indicating which system is used in the patient's pathology and clinical reports. Eur Urol. 2010 Sep;58(3):369-73. doi: 10.1016/j.eururo.2010.04.028. Epub 2010 May 8. PMID: 20478652.
- Epstein JI, Egevad L, Amin MB, Delahunt B, Srigley JR, Humphrey PA; Grading Committee. The 2014 International Society of Urological Pathology (ISUP) Consensus Conference on Gleason Grading of Prostatic Carcinoma: Definition of Grading Patterns and Proposal for a New Grading System. Am J Surg Pathol. 2016 Feb;40(2):244-52. doi: 10.1097/PAS.0000000000000530. PMID: 26492179.
- Pudasaini S, Subedi N. Understanding the gleason grading system and its changes. J Path Nepal. 2019;9(2):1580-1585. doi 10.3126/jpn.v9i2.25723.
- Ström P, Kartasalo K, Olsson H, Solorzano L, Delahunt B, Berney DM, Bostwick DG, Evans AJ, Grignon DJ, Humphrey PA, Iczkowski KA, Kench JG, Kristiansen G, van der Kwast TH, Leite KRM, McKenney JK, Oxley J, Pan CC, Samaratunga H, Srigley JR, Takahashi H, Tsuzuki T, Varma M, Zhou M, Lindberg J, Lindskog C, Ruusuvuori P, Wählby C, Grönberg H, Rantalainen M, Egevad L, Eklund M. Artificial intelligence for diagnosis and grading of prostate cancer in biopsies: a population-based, diagnostic study. Lancet Oncol. 2020 Feb;21(2):222-232. doi: 10.1016/S1470-2045(19)30738-7. Epub 2020 Jan 8. Erratum in: Lancet Oncol. 2020 Feb;21(2):e70. PMID: 31926806.
- Nagpal K, Foote D, Tan F, Liu Y, Chen PC, Steiner DF, Manoj N, Olson N, Smith JL, Mohtashamian A, Peterson B, Amin MB, Evans AJ, Sweet JW, Cheung C, van der Kwast T, Sangoi AR, Zhou M, Allan R, Humphrey PA, Hipp JD, Gadepalli K, Corrado GS, Peng LH, Stumpe MC, Mermel CH. Development and Validation of a Deep Learning Algorithm for Gleason Grading of Prostate Cancer From Biopsy Specimens. JAMA Oncol. 2020 Sep 1;6(9):1372-1380. doi: 10.1001/jamaoncol.2020.2485. PMID: 32701148; PMCID: PMC7378872.
- Bulten W, Kartasalo K, Chen PC, Ström P, Pinckaers H, Nagpal K, Cai Y, Steiner DF, van Boven H, Vink R, Hulsbergen-van de Kaa C, van der Laak J, Amin MB, Evans AJ, van der Kwast T, Allan R, Humphrey PA, Grönberg H, Samaratunga H, Delahunt B, Tsuzuki T, Häkkinen T, Egevad L, Demkin M, Dane S, Tan F, Valkonen M, Corrado GS, Peng L, Mermel CH, Ruusuvuori P, Litjens G, Eklund M; PANDA challenge consortium. Artificial intelligence for diagnosis and Gleason grading of prostate cancer: the PANDA challenge. Nat Med. 2022 Jan;28(1):154-163. doi: 10.1038/s41591-021-01620-2. Epub 2022 Jan 13. PMID: 35027755; PMCID: PMC8799467.
- Spivak DI. Category theory for the sciences. Cambridge: The MIT Presss; 2014. p.203-314.
- Rikkers LF. The bandwagon effect. J Gastrointest Surg. 2002 Nov-Dec;6(6):787-94. doi: 10.1016/s1091-255x(02)00054-9. PMID: 12504215.
- Cohen L, Rothschild H. The bandwagons of medicine. Perspect Biol Med. 1979 Summer;22(4):531-8. doi: 10.1353/pbm.1979.0037. PMID: 226929.
- Li W, Li J, Sarma KV, Ho KC, Shen S, Knudsen BS, Gertych A, Arnold CW. Path R-CNN for Prostate Cancer Diagnosis and Gleason Grading of Histological Images. IEEE Trans Med Imaging. 2019 Apr;38(4):945-954. doi: 10.1109/TMI.2018.2875868. Epub 2018 Oct 12. PMID: 30334752; PMCID: PMC6497079.
- Gödel K. Über formal unentscheidbare Sätze der Principia Mathematica und verwandter Systeme, I", Monatshefte für Mathematik und Physik, 1931;38(1):173–198. doi:10.1007/BF01700692.
- Gödel K. Some basic theorems on the foundations of mathematics and their implications. In: Feferman S, editor. Kurt Gödel Collected works. Vol. III, Oxford University Press; 1995. p.304-323.
- Heaven D. Why deep-learning AIs are so easy to fool. Nature. 2019 Oct;574(7777):163-166. doi: 10.1038/d41586-019-03013-5. PMID: 31597977.
- Colbrook MJ, Antun V, Hansen AC. The difficulty of computing stable and accurate neural networks: On the barriers of deep learning and Smale's 18th problem. Proc Natl Acad Sci U S A. 2022 Mar 22;119(12):e2107151119. doi: 10.1073/pnas.2107151119. Epub 2022 Mar 16. PMID: 35294283; PMCID: PMC8944871.
- Sethna JP. Statistical mechanics. Entropy, order parameters, and complexity. Oxford: Clarendon Press. 2011;101-102. doi:10.1093/oso/9780198865247.001.0001.
- Kristiansen G, Epstein JI. Immunohistochemistry in prostate pathology. IHC Prostate Pathology. DAKO. 2013.
- Salat H, Murcio R, Arcaute E. Multifractal methodology. Physica A. Statistical Mechanics and its applications. 2017; 473:467-487. doi.10.1016/j.physa.2017.01.041.
- Eckmann JP, Kamphorst SO, Ruelle D. Recurrence plots of dynamical systems. Europhys Letter. 1987;4(9):973-977. doi:10.1209/0295-5075/4/9/004.
- Takens F. Detecting strange attractors in turbulence. In: Rand D, Young LS, editors. Dynamical Systems and Turbulence. Lecture Notes in Mathematics. Berlin, Heidelberg:Springer. 1980. doi: 10.1007/BFb0091924.
- Morris SA. Topology without tears; 2020. p.25.
- Munkres J. Topology. Prentice Hall; 2000. p.148.
- Gamelin TW, Greene RE. Introduction to topology. Dover Publications; 1999. p.67
- Zomorodian A, Carlsson GE. Computing persistent homology. Discrete Computational Geometry. 2005;33(2):249-274. doi.10.1007/s00454-004-1146-y.
- Morozov D, Edelsbrunner H. A practical guide to persistent homology. Lawrence Berkeley National Lab. 2022.
- Lawson P, Sholl AB, Brown JQ, Fasy BT, Wenk C. Persistent Homology for the Quantitative Evaluation of Architectural Features in Prostate Cancer Histology. Sci Rep. 2019 Feb 4;9(1):1139. doi: 10.1038/s41598-018-36798-y. PMID: 30718811; PMCID: PMC6361896.
- Kerber M, Morozov D, Nigmetov A. Geometry helps to compare persistence diagrams. J Exp Algorithmics. 2017;22(1):1-20. doi:10.1145/3064175.
- Kerber M, Lesnick M, Oudot S. Exact computation of the matching distance on 2-parameter persistence modules. JoCG. 2020;11(2):4-25.
- Munch, E. Application of persistent homology to time varying systems. Duke University. 2013.
- Saul N, Tralie Ch. Scikit-TDA: Topological data analysis for python. Zenodo. 2019. doi: 10.5281/zenodo.2533369.
- Waliszewski P, Banaszak G. Topological evaluation of intercellular connectivity in adenocarcinomas. Proceedings of the 23rd International Conference on Control Systems and Computer Science (CSCS21). 2021. doi: 10.1109/CSCS52396.2021.00042.
- Levin M. Bioelectric signaling: Reprogrammable circuits underlying embryogenesis, regeneration, and cancer. Cell. 2021 Apr 15;184(8):1971-1989. doi: 10.1016/j.cell.2021.02.034. Epub 2021 Apr 6. PMID: 33826908.
- Carvalho J. A computational model of cell membrane bioelectric polarization and depolarization, connected with cell proliferation, in different tissue geometries. J Theor Biol. 2023 Jan 21;557:111338. doi: 10.1016/j.jtbi.2022.111338. Epub 2022 Nov 4. PMID: 36343668.
- Bates E. Ion channels in development and cancer. Annu Rev Cell Dev Biol. 2015;31:231-47. doi: 10.1146/annurev-cellbio-100814-125338. PMID: 26566112.
- Tanase M, Waliszewski P. On Complexity and homogenity measures in predicting biological aggressiveness of prostate cancer; implication of the cellular automata model of tumor growth. Journal of Surgical Oncology. 2015;112(8):791-801. doi: 10.1002/jso.24069.
- Waliszewski P. The circular fractal model of adenocarcinomas and tumor aggressiveness. Banach Center Publications. 2016;109:183-196. doi: 10.4064/bc109-0-12.
- Waliszewski P. The Fibonacci constant and limits of tissue self-organization; local complexity measures in evaluation of the risk of metastasis formation. Banach Center Publications. 2021;124:143-157. doi: 10.4064/bc124-12.
- Waliszewski P, Konarski J, Molski M. On the modification of fractal self-space during tumor progression. Fractals 2000;8(2):195-203. doi: 10.1142/S0218348X00000214.
- Waliszewski P. The Fibonacci constant, the Wasserstein distance, and biological tumor aggressiveness in prostate cancer. Proceedings of the 24th International Conference on Control Systems and Computer Science (CSCS23). 2023. doi: 10.1109/CSCS59211.2023.00043.
- Adams H, Aminian M, Farnell E, Kirby M, Peterson Ch, Mirth J, Neville R, Shipman P, Shonkwiler C. A fractal dimension for measures via persistent homology. In: Baas N, Carlsson G, Quick G, Szymik M, Thaule M, editors. Topological Data Analysis. Abel Symposia. 2020;15:1-31. Springer, Cham. doi: 10.1007/978-3-030-43408-3_1.
- Nicolau M, Levine AJ, Carlsson G. Topology based data analysis identifies a subgroup of breast cancers with a unique mutational profile and excellent survival. Proc Natl Acad Sci U S A. 2011 Apr 26;108(17):7265-70. doi: 10.1073/pnas.1102826108. Epub 2011 Apr 11. PMID: 21482760; PMCID: PMC3084136.
- Nowak MA. Five rules for the evolution of cooperation. Science. 2006 Dec 8;314(5805):1560-3. doi: 10.1126/science.1133755. PMID: 17158317; PMCID: PMC3279745.
- Fozard JA, Kirkham GR, Buttery LD, King JR, Jensen OE, Byrne HM. Techniques for analysing pattern formation in populations of stem cells and their progeny. BMC Bioinformatics. 2011 Oct 12;12:396. doi: 10.1186/1471-2105-12-396. PMID: 21991994; PMCID: PMC3252362.
- Nelson CM, Jean RP, Tan JL, Liu WF, Sniadecki NJ, Spector AA, Chen CS. Emergent patterns of growth controlled by multicellular form and mechanics. Proc Natl Acad Sci U S A. 2005 Aug 16;102(33):11594-9. doi: 10.1073/pnas.0502575102. Epub 2005 Jul 27. PMID: 16049098; PMCID: PMC1187971.
- Snyder JC, Rochelle LK, Marion S, Lyerly HK, Barak LS, Caron MG. Lgr4 and Lgr5 drive the formation of long actin-rich cytoneme-like membrane protrusions. J Cell Sci. 2015 Mar 15;128(6):1230-40. doi: 10.1242/jcs.166322. Epub 2015 Feb 4. PMID: 25653388; PMCID: PMC4359926.
- Imran Alsous J, Villoutreix P, Berezhkovskii AM, Shvartsman SY. Collective Growth in a Small Cell Network. Curr Biol. 2017 Sep 11;27(17):2670-2676.e4. doi: 10.1016/j.cub.2017.07.038. Epub 2017 Aug 31. PMID: 28867205; PMCID: PMC6333208.
- Mandal M, Ghosh B, Rajput M, Chatterjee J. Impact of intercellular connectivity on epithelial mesenchymal transition plasticity. Biochim Biophys Acta Mol Cell Res. 2020 Oct;1867(10):118784. doi: 10.1016/j.bbamcr.2020.118784. Epub 2020 Jun 23. PMID: 32590027.
- Waliszewski P. Computer-Aided Image Analysis and Fractal Synthesis in the Quantitative Evaluation of Tumor Aggressiveness in Prostate Carcinomas. Front Oncol. 2016 May 9;6:110. doi: 10.3389/fonc.2016.00110. PMID: 27242954; PMCID: PMC4860606.