Oivind Riis*, Andreas Stensvold, Helge Stene-Johansen and Frank Westad
Volume4-Issue6
Dates: Received: 2023-06-06 | Accepted: 2023-06-13 | Published: 2023-06-14
Pages: 1030-1038
Abstract
Introduction: We report in this study the results of analyzing biomarkers in blood samples with two objectives; i) as an approach for screening patients by use of Multivariate Statistical Process Control (MSPC); ii) Compare various classification methods with the purpose of diagnosing prostate cancer.
Methods: We applied Principal Component Analysis (PCA) with statistical limits for outlier detection. Various splits of the data into training and test sets were chosen to evaluate the performance of classification methods as a function of the training/test sample ratio.
Results: MSPC based on 12 analytes in blood samples was shown to outperform the traditional biomarker criterion: the level of the analyte Prostate-Specific Antigen (PSA), in screening for prostate cancer. The performance of different multivariate classification techniques for classifying which of the patients in a clinical pathway for prostate cancer have malignant tumors showed that the basic method Linear Discriminant Analysis (LDA) and classification trees gave similar results, whereas adaboost gave a higher specificity but lower sensitivity.
Conclusion: The accuracy, especially the sensitivity, does not justify any clinical use of the applied classification methods with the available biomarkers. Additional medical information about the patients might enhance the accuracy with the purpose of identifying benign and malignant tumors.
FullText HTML
FullText PDF
DOI: 10.37871/jbres1764
Certificate of Publication
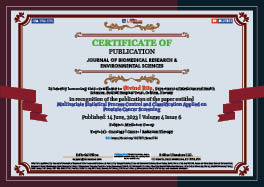
Copyright
© 2023 Riis Ø, et al. Distributed under Creative Commons CC-BY 4.0
How to cite this article
Riis Ø, Stensvold A, Stene-Johansen H, Westad F. Multivariate Statistical Process Control and Classifi cation Applied on Prostate Cancer Screening. 2023 June 14; 4(6): 1030-1038. doi: 10.37871/jbres1764, Article ID: JBRES1764, Available at: https://www.jelsciences.com/articles/jbres1764.pdf
Subject area(s)
References
- Medipally DKR, Cullen D, Untereiner V, Sockalingum GD, Maguire A, Nguyen TNQ, Bryant J, Noone E, Bradshaw S, Finn M, Dunne M, Shannon AM, Armstrong J, Meade AD, Lyng FM. Vibrational spectroscopy of liquid biopsies for prostate cancer diagnosis. Ther Adv Med Oncol. 2020 Jul 30;12:1758835920918499. doi: 10.1177/1758835920918499. PMID: 32821294; PMCID: PMC7412923.
- Martynko E, Oleneva E, Andreev E, Savinov S, Solovieva S, Protoshchak V, Karpushchenko E, Sleptsov A, Panchuk V, Legin A, Kirsanov D. Non-invasive prostate cancer screening using chemometric processing of macro and trace element concentration profiles in urine. Microchemical Journal. 2020;159:105464. doi: 10.1016/j.microc.2020.105464.
- Su KY, Lee WL. Fourier Transform Infrared Spectroscopy as a Cancer Screening and Diagnostic Tool: A Review and Prospects. Cancers (Basel). 2020 Jan 1;12(1):115. doi: 10.3390/cancers12010115. PMID: 31906324; PMCID: PMC7017192.
- Amante E, Salomone A, Alladio E, Vincenti M, Porpiglia F, Bro R. Untargeted Metabolomic Profile for the Detection of Prostate Carcinoma-Preliminary Results from PARAFAC2 and PLS-DA Models. Molecules. 2019 Aug 22;24(17):3063. doi: 10.3390/molecules24173063. PMID: 31443574; PMCID: PMC6749415.
- Lubes G, Goodarzi M. GC-MS based metabolomics used for the identification of cancer volatile organic compounds as biomarkers. J Pharm Biomed Anal. 2018 Jan 5;147:313-322. doi: 10.1016/j.jpba.2017.07.013. Epub 2017 Jul 17. PMID: 28750734.
- Guo J, Zhang X, Xia T, Johnson H, Feng X, Simoulis A, Wu AHB, Li F, Tan W, Johnson A, Dizeyi N, Abrahamsson PA, Kenner L, Xiao K, Zhang H, Chen L, Zou C, Persson JL. Non-invasive Urine Test for Molecular Classification of Clinical Significance in Newly Diagnosed Prostate Cancer Patients. Front Med (Lausanne). 2021 Sep 14;8:721554. doi: 10.3389/fmed.2021.721554. PMID: 34595190; PMCID: PMC8476767.
- Solovieva S, Karnaukh M, Panchuk V, Andreev E, Kartsova L, Bessonova E, Legin A, Wang P, Wan H, Jahatspanian I, Kirsanov D. Potentiometric multisensor system as a possible simple tool for non-invasive prostate cancer diagnostics through urine analysis. Sensors and Actuators B: Chemical. 2019;289:42-47. doi: 10.1016/j.snb.2019.03.072.
- Aggio RB, de Lacy Costello B, White P, Khalid T, Ratcliffe NM, Persad R, Probert CS. The use of a gas chromatography-sensor system combined with advanced statistical methods, towards the diagnosis of urological malignancies. J Breath Res. 2016 Feb 11;10(1):017106. doi: 10.1088/1752-7155/10/1/017106. PMID: 26865331; PMCID: PMC4876927.
- Vergel ÁJS, Mendoza LE, Delgado BM. Analysis of energy and major components in chromatographic signals for the diagnosis of prostate cancer. Respuestas. 2019;24:76-85.
- Ljubicic ML, Madsen A, Juul A, Almstrup K, Johannsen TH. The Application of Principal Component Analysis on Clinical and Biochemical Parameters Exemplified in Children With Congenital Adrenal Hyperplasia. Front Endocrinol (Lausanne). 2021 Aug 31;12:652888. doi: 10.3389/fendo.2021.652888. PMID: 34531821; PMCID: PMC8438425.
- Jackson JE. A user’s guide to principal components. John Wiley & Sons; 2005.
- Ge Z, Song Z. Multivariate statistical process control: Process monitoring methods and applications. Springer Science & Business Media; 2012.
- Solberg HE. Editorial: Discriminant analysis in clinical chemistry. Scand J Clin Lab Invest. 1975 Dec;35(8):705-12. doi: 10.3109/00365517509095801. PMID: 1108173.
- Corte C, Vapnik V. Support vector machines. Machine Learning. 1995;20:273-297. doi: 10.1007/bf00994018.
- Breiman L, Friedman JH, Olshen RA, Stone CJ. Classification and Regression Trees. Routledge; 2017.
- Schapire RE. Explaining adaboost. In: Empirical Inference. Springer; 2013. p.37-52.
- Westad F, Marini F. Validation of chemometric models - a tutorial. Anal Chim Acta. 2015 Sep 17;893:14-24. doi: 10.1016/j.aca.2015.06.056. Epub 2015 Aug 10. PMID: 26398418.
- Brain D, Webb GI. The need for low bias algorithms in classification learning from large data sets. In Proceedings of the European Conference on Principles of Data Mining and Knowledge Discovery. Springer; 2002. p.62-73.
- Kennard RW, Stone LA. Computer aided design of experiments. Technometrics. 1969;11:137-148. doi: 10.2307/1266770
- Putra IB, Hamid AR, Mochtar CA, Umbas R. Relationship of age, prostate-specific antigen, and prostate volume in Indonesian men with benign prostatic hyperplasia. Prostate Int. 2016 Jun;4(2):43-8. doi: 10.1016/j.prnil.2016.03.002. Epub 2016 Mar 11. PMID: 27358842; PMCID: PMC4916066.