Abeer Alzubaidi, Jonathan Tepper, Benjamin Inden and Ahmad Lotfi*
Volume3-Issue10
Dates: Received: 2022-09-27 | Accepted: 2022-10-11 | Published: 2022-10-13
Pages: 1163-1176
Abstract
Early detection of breast cancer and effective identification of its correct stage remain major challenges for healthcare professionals. Testing the tumour for Oestrogen Receptor and Progesterone Receptor is a standard part of the initial evaluation of breast cancer diagnosis and treatment planning. Several expression profiling studies have illustrated that the expression of these hormone receptors is linked with diverse genetic variations, which means that several mutated genes can a affect the development and progression of breast cancer and contribute to its heterogeneity. Unfortunately, due to the high dimensionality and low sample size nature of microarray data, traditional statistical feature selection techniques fail to identify genes that could act as risk factors for breast cancer. Inspired by this, we developed a deep learning-based feature extraction module with a weight interpretation method to select a subset of robust biomarkers across three different mRNA expression data sets from The Cancer Genome Atlas program (TCGA). For a discovered feature (a gene) to be accepted for further investigation, it must have been independently selected by the weight interpretation method from each of the deep feature extraction modules (each having been trained on a different data set). The small panel of discovered biomarkers was then subsequently evaluated using a range of classifiers to ascertain their predictive ability with respect to the above hormone receptor status. We observed strong evidence that the upregulation in the expression levels of highly positively weighted genes within the deep feature selection modules and the down regulation in the expression levels of the highly negatively weighted genes both indicated the strong likelihood of a patient experiencing ER+/PR+ invasive breast cancer. In addition, we discovered a number of potentially novel biomarkers worthy of further consideration.
FullText HTML
FullText PDF
DOI: 10.37871/jbres1572
Certificate of Publication
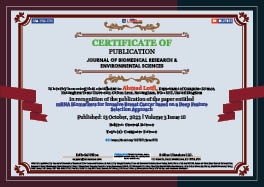
Copyright
© 2022 Alzubaidi A, et al. Distributed under Creative Commons CC-BY 4.0
How to cite this article
Alzubaidi A, Tepper J, Inden B, Lotfi A. mRNA Biomarkers for Invasive Breast Cancer based on a Deep Feature Selection Approach. 2022 Oct 13; 3(10): 1163-1176. doi: 10.37871/jbres1572, Article ID: JBRES1572, Available at: https://www.jelsciences.com/articles/jbres1572.pdf
Subject area(s)
References
- Hamid Behravan, Jaana M Hartikainen, Maria Tengstr¨om, Katri Pylk¨as, Robert Winqvist, Veli-Matti Kosma, and Arto Mannermaa. Machine learning identifies interacting genetic variants contributing to breast can- cer risk: A case study in finnish cases and controls. Scientific reports. 2018;8(1):13149.
- Ruth Etzioni, Nicole Urban, Scott Ramsey, Martin McIntosh, Stephen Schwartz, Brian Reid, Jerald Radich, Garnet Anderson, and Leland Hartwell. Early detection: The case for early detection. Nature Reviews Cancer. 2003;3(4):243.
- Gøtzsche PC, Nielsen M. Screening for breast cancer with mammography. Cochrane Database Syst Rev. 2011 Jan 19;(1):CD001877. doi: 10.1002/14651858.CD001877.pub4. Update in: Cochrane Database Syst Rev. 2013;6:CD001877. PMID: 21249649.
- Welch HG, Prorok PC, O'Malley AJ, Kramer BS. Breast-Cancer Tumor Size, Overdiagnosis, and Mammography Screening Effectiveness. N Engl J Med. 2016 Oct 13;375(15):1438-1447. doi: 10.1056/NEJMoa1600249. PMID: 27732805.
- Berg WA. Tailored supplemental screening for breast cancer: what now and what next? AJR Am J Roentgenol. 2009 Feb;192(2):390-9. doi: 10.2214/AJR.08.1706. PMID: 19155400.
- Hooley RJ, Andrejeva L, Scoutt LM. Breast cancer screening and problem solving using mammography, ultrasound, and magnetic resonance imaging. Ultrasound Q. 2011 Mar;27(1):23-47. doi: 10.1097/RUQ.0b013e31820e15ac. PMID: 21343800.
- Hammond ME, Hayes DF, Dowsett M, Allred DC, Hagerty KL, Badve S, Fitzgibbons PL, Francis G, Goldstein NS, Hayes M, Hicks DG, Lester S, Love R, Mangu PB, McShane L, Miller K, Osborne CK, Paik S, Perlmutter J, Rhodes A, Sasano H, Schwartz JN, Sweep FC, Taube S, Torlakovic EE, Valenstein P, Viale G, Visscher D, Wheeler T, Williams RB, Wittliff JL, Wolff AC; American Society of Clinical Oncology; College of American Pathologists. American Society of Clinical Oncology/College of American Pathologists guideline recommendations for immunohistochemical testing of estrogen and progesterone receptors in breast cancer (unabridged version). Arch Pathol Lab Med. 2010 Jul;134(7):e48-72. doi: 10.5858/134.7.e48. PMID: 20586616.
- Benita S Katzenellenbogen, Monica M Montano, Kirk Ekena, Mary E Herman, and Eileen M McInerney. Antiestrogens: mechanisms of action and resistance in breast cancer. Breast cancer research and treatment. 1997;44(1):23-38.
- Shiau AK, Barstad D, Loria PM, Cheng L, Kushner PJ, Agard DA, Greene GL. The structural basis of estrogen receptor/coactivator recognition and the antagonism of this interaction by tamoxifen. Cell. 1998 Dec 23;95(7):927-37. doi: 10.1016/s0092-8674(00)81717-1. PMID: 9875847.
- Carroll JS. Mechanisms of oestrogen receptor (ER) gene regulation in breast cancer. European journal of endocrinology. 2016;175(1):R41–9.
- Sorlie T, Tibshirani R, Parker J, Hastie T, Marron JS, Nobel A, Deng S, Johnsen H, Pesich R, Geisler S, Demeter J, Perou CM, Lønning PE, Brown PO, Børresen-Dale AL, Botstein D. Repeated observation of breast tumor subtypes in independent gene expression data sets. Proc Natl Acad Sci U S A. 2003 Jul 8;100(14):8418-23. doi: 10.1073/pnas.0932692100. Epub 2003 Jun 26. PMID: 12829800; PMCID: PMC166244.
- Christof Angermueller, Heather Lee, Wolf Reik, and Oliver Stegle. Accu- rate prediction of single-cell dna methylation states using deep learning. BioRxiv. 2017;055715.
- Kelley DR, Snoek J, Rinn JL. Basset: learning the regulatory code of the accessible genome with deep convolutional neural networks. Genome Res. 2016 Jul;26(7):990-9. doi: 10.1101/gr.200535.115. Epub 2016 May 3. PMID: 27197224; PMCID: PMC4937568.
- Patel-Murray NL, Adam M, Huynh N, Wassie BT, Milani P, Fraenkel E. A Multi-Omics Interpretable Machine Learning Model Reveals Modes of Action of Small Molecules. Sci Rep. 2020 Jan 22;10(1):954. doi: 10.1038/s41598-020-57691-7. PMID: 31969612; PMCID: PMC6976599.
- Zhou J, Troyanskaya OG. Predicting effects of noncoding variants with deep learning-based sequence model. Nat Methods. 2015 Oct;12(10):931-4. doi: 10.1038/nmeth.3547. Epub 2015 Aug 24. PMID: 26301843; PMCID: PMC4768299.
- David E Rumelhart and James L McClelland. Parallel distributed pro- cessing: explorations in the microstructure of cognition. 1986.
- Ian Goodfellow, Yoshua Bengio, and Aaron Courville. Deep learning. MIT press. 2016.
- Abeer Alzubaidi and Jonathan Tepper. Deep mining from omics data. In Data Mining Techniques for the Life Sciences. Humana, New York, 2022.
- Alzubaidi A, Tepper J, Lotfi A. A novel deep mining model for effective knowledge discovery from omics data. Artif Intell Med. 2020 Apr;104:101821. doi: 10.1016/j.artmed.2020.101821. Epub 2020 Feb 24. PMID: 32499000.
- Taylor JM, Ankerst DP, Andridge RR. Validation of biomarker-based risk prediction models. Clin Cancer Res. 2008 Oct 1;14(19):5977-83. doi: 10.1158/1078-0432.CCR-07-4534. PMID: 18829476; PMCID: PMC3896456.
- Cancer Genome Atlas Research Network, Weinstein JN, Collisson EA, Mills GB, Shaw KR, Ozenberger BA, Ellrott K, Shmulevich I, Sander C, Stuart JM. The Cancer Genome Atlas Pan-Cancer analysis project. Nat Genet. 2013 Oct;45(10):1113-20. doi: 10.1038/ng.2764. PMID: 24071849; PMCID: PMC3919969.
- Gao J, Aksoy BA, Dogrusoz U, Dresdner G, Gross B, Sumer SO, Sun Y, Jacobsen A, Sinha R, Larsson E, Cerami E, Sander C, Schultz N. Integrative analysis of complex cancer genomics and clinical profiles using the cBioPortal. Sci Signal. 2013 Apr 2;6(269):pl1. doi: 10.1126/scisignal.2004088. PMID: 23550210; PMCID: PMC4160307.
- Isaac S Kohane, Atul J Butte, and Alvin Kho. Microarrays for an integrative genomics. MIT press, 2002.
- Cancer Genome Atlas Network. Comprehensive molecular portraits of human breast tumours. Nature. 2012 Oct 4;490(7418):61-70. doi: 10.1038/nature11412. Epub 2012 Sep 23. PMID: 23000897; PMCID: PMC3465532.
- Ciriello G, Gatza ML, Beck AH, Wilkerson MD, Rhie SK, Pastore A, Zhang H, McLellan M, Yau C, Kandoth C, Bowlby R, Shen H, Hayat S, Fieldhouse R, Lester SC, Tse GM, Factor RE, Collins LC, Allison KH, Chen YY, Jensen K, Johnson NB, Oesterreich S, Mills GB, Cherniack AD, Robertson G, Benz C, Sander C, Laird PW, Hoadley KA, King TA; TCGA Research Network, Perou CM. Comprehensive Molecular Portraits of Invasive Lobular Breast Cancer. Cell. 2015 Oct 8;163(2):506-19. doi: 10.1016/j.cell.2015.09.033. PMID: 26451490; PMCID: PMC4603750.
- Zheng Ping, Yuchao Xia, Tiansheng Shen, Vishwas Parekh, Gene P Siegal, Isam-Eldin Eltoum, Jianbo He, Dongquan Chen, Minghua Deng, Ruibin Xi, et al. A microscopic landscape of the invasive breast cancer genome. Scientific reports. 2016;6:27545.
- Martin Fodslette Møller. A scaled conjugate gradient algorithm for fast supervised learning. Neural networks. 1993;6(4):525-533.
- Bernhard E Boser, Isabelle M Guyon, and Vladimir N Vapnik. A training algorithm for optimal margin classifiers. In Proceedings of the fifth annual workshop on Computational learning theory. ACM. 1992;144–152.
- Leo Breiman. Bagging predictors. Machine learning. 1996;24(2):123-140.
- Reinert T, Gonçalves R, Bines J. Implications of ESR1 Mutations in Hormone Receptor-Positive Breast Cancer. Curr Treat Options Oncol. 2018 Apr 17;19(5):24. doi: 10.1007/s11864-018-0542-0. PMID: 29666928.
- Fielder GC, Yang TW, Razdan M, Li Y, Lu J, Perry JK, Lobie PE, Liu DX. The GDNF Family: A Role in Cancer? Neoplasia. 2018 Jan;20(1):99-117. doi: 10.1016/j.neo.2017.10.010. Epub 2017 Dec 12. PMID: 29245123; PMCID: PMC5730419.
- Garczyk S, von Stillfried S, Antonopoulos W, Hartmann A, Schrauder MG, Fasching PA, Anzeneder T, Tannapfel A, Ergönenc Y, Knüchel R, Rose M, Dahl E. AGR3 in breast cancer: prognostic impact and suitable serum-based biomarker for early cancer detection. PLoS One. 2015 Apr 15;10(4):e0122106. doi: 10.1371/journal.pone.0122106. PMID: 25875093; PMCID: PMC4398490.
- van der Willik KD, Timmermans MM, van Deurzen CH, Look MP, Reijm EA, van Zundert WJ, Foekens R, Trapman-Jansen AM, den Bakker MA, Westenend PJ, Martens JW, Berns EM, Jansen MP. SIAH2 protein expression in breast cancer is inversely related with ER status and outcome to tamoxifen therapy. Am J Cancer Res. 2016 Jan 15;6(2):270-84. PMID: 27186402; PMCID: PMC4859659.
- Carlisle SM, Hein DW. Retrospective analysis of estrogen receptor 1 and N acetyltransferase gene expression in normal breast tissue, primary breast tumors, and established breast cancer cell lines. Int J Oncol. 2018 Aug;53(2):694-702. doi: 10.3892/ijo.2018.4436. Epub 2018 Jun 11. PMID: 29901116; PMCID: PMC6017241.
- Lin YC, Lee YC, Li LH, Cheng CJ, Yang RB. Tumor suppressor SCUBE2 inhibits breast-cancer cell migration and invasion through the reversal of epithelial-mesenchymal transition. J Cell Sci. 2014 Jan 1;127(Pt 1):85-100. doi: 10.1242/jcs.132779. Epub 2013 Nov 8. PMID: 24213532.
- Takaku M, Grimm SA, Roberts JD, Chrysovergis K, Bennett BD, Myers P, Perera L, Tucker CJ, Perou CM, Wade PA. GATA3 zinc finger 2 mutations reprogram the breast cancer transcriptional network. Nat Commun. 2018 Mar 13;9(1):1059. doi: 10.1038/s41467-018-03478-4. PMID: 29535312; PMCID: PMC5849768.
- Willis S, Sun Y, Abramovitz M, Fei T, Young B, Lin X, Ni M, Achua J, Regan MM, Gray KP, Gray R, Wang V, Long B, Kammler R, Sparano JA, Williams C, Goldstein LJ, Salgado R, Loi S, Pruneri G, Viale G, Brown M, Leyland-Jones B. High Expression of FGD3, a Putative Regulator of Cell Morphology and Motility, Is Prognostic of Favorable Outcome in Multiple Cancers. JCO Precis Oncol. 2017 Oct 13;1:PO.17.00009. doi: 10.1200/PO.17.00009. PMID: 32913979; PMCID: PMC7446538.
- Moy I, Todorović V, Dubash AD, Coon JS, Parker JB, Buranapramest M, Huang CC, Zhao H, Green KJ, Bulun SE. Estrogen-dependent sushi domain containing 3 regulates cytoskeleton organization and migration in breast cancer cells. Oncogene. 2015 Jan 15;34(3):323-33. doi: 10.1038/onc.2013.553. Epub 2014 Jan 13. PMID: 24413080; PMCID: PMC4096609.
- Morgat C, MacGrogan G, Brouste V, Vélasco V, Sévenet N, Bonnefoi H, Fernandez P, Debled M, Hindié E. Expression of Gastrin-Releasing Peptide Receptor in Breast Cancer and Its Association with Pathologic, Biologic, and Clinical Parameters: A Study of 1,432 Primary Tumors. J Nucl Med. 2017 Sep;58(9):1401-1407. doi: 10.2967/jnumed.116.188011. Epub 2017 Mar 9. PMID: 28280221.
- Hertz DL, Henry NL, Kidwell KM, Thomas D, Goddard A, Azzouz F, Speth K, Li L, Banerjee M, Thibert JN, Kleer CG, Stearns V, Hayes DF, Skaar TC, Rae JM. ESR1 and PGR polymorphisms are associated with estrogen and progesterone receptor expression in breast tumors. Physiol Genomics. 2016 Sep 1;48(9):688-98. doi: 10.1152/physiolgenomics.00065.2016. Epub 2016 Aug 19. PMID: 27542969; PMCID: PMC5111879.
- Cheng M, Michalski S, Kommagani R. Role for Growth Regulation by Estrogen in Breast Cancer 1 (GREB1) in Hormone-Dependent Cancers. Int J Mol Sci. 2018 Aug 28;19(9):2543. doi: 10.3390/ijms19092543. PMID: 30154312; PMCID: PMC6163654.
- Segaert P, Lopes MB, Casimiro S, Vinga S, Rousseeuw PJ. Robust identification of target genes and outliers in triple-negative breast cancer data. Stat Methods Med Res. 2019 Oct-Nov;28(10-11):3042-3056. doi: 10.1177/0962280218794722. Epub 2018 Aug 27. PMID: 30146936; PMCID: PMC6745616.
- Zhang Y, He W, Zhang S. Seeking for Correlative Genes and Signaling Pathways With Bone Metastasis From Breast Cancer by Integrated Analysis. Front Oncol. 2019 Mar 13;9:138. doi: 10.3389/fonc.2019.00138. PMID: 30918839; PMCID: PMC6424882.
- Gao S, Ge A, Xu S, You Z, Ning S, Zhao Y, Pang D. PSAT1 is regulated by ATF4 and enhances cell proliferation via the GSK3β/β-catenin/cyclin D1 signaling pathway in ER-negative breast cancer. J Exp Clin Cancer Res. 2017 Dec 8;36(1):179. doi: 10.1186/s13046-017-0648-4. PMID: 29216929; PMCID: PMC5721480.