Ligia Flavia A Batista*, Fernanda SY Watanabe, Luiz Henrique S Rotta and Nilton N Imai
Volume3-Issue9
Dates: Received: 2022-09-08 | Accepted: 2022-09-13 | Published: 2022-09-16
Pages: 1057-1064
Abstract
In some tropical reservoirs, aquatic macrophytes have covered extensive areas and it is difficult to monitor, control and forecast their proliferation. The major issues are economic and ecological because macrophyte growth has caused significant financial losses to hydroelectric power plants and has affected the ecological balance. Thus, this work aimed to infer the most likely areas to be colonized by Submerged Aquatic Vegetation (SAV), using influencing factors, such as the morphometric aspects of the area and any preexisting vegetation, to support the management decision-making process. Four field surveys were carried out to collect hydroacoustic data, which indicate presence of SAV, in Taquaruçu reservoir, Paranapanema River, Brazil. The inference procedure was applied using the weights of evidence method. The results showed that depths up to 6 m have a high probability of colonization and that points with five or six colonized neighbor’s cells, considering a regular grid, are also more likely to be colonized. The weights for the slope map behaved differently at each survey interval. The probability maps of colonization were generated and can be very useful in supporting the decision-making process of SAV management activities, such as concentrating the monitoring efforts for the high-probability colonization areas, in this case those which the lowest depths or next to colonized regions.
FullText HTML
FullText PDF
DOI: 10.37871/jbres1555
Certificate of Publication
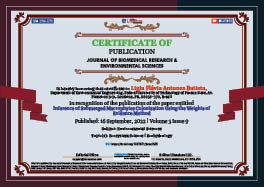
Copyright
© 2022 Batista LFA, et al. Distributed under Creative Commons CC-BY 4.0
How to cite this article
How to cite this article: Batista LFA, Watanabe FSY, Rotta LHS, Imai NN. Inference of Submerged Macrophytes Colonization Using the Weights of Evidence Method. J Biomed Res Environ Sci. 2022 Sep 16; 3(9): 1057-1064. doi: 10.37871/jbres1555, Article ID: JBRES1555, Available at: https://www.jelsciences.com/articles/jbres1555.pdf
Subject area(s)
References
- Silva TSF, Costa MPF, Melack JM. Assessment of two biomass estimation methods for aquatic vegetation growing on the Amazon Floodplain. Aquat Bot. 2010;92(3):161-167. doi: 10.1016/j.aquabot.2009.10.015.
- Thomaz SM, Esteves FA. Comunidade de Macrófitas Aquáticas. [Aquatic Macrophytes Community] In: Esteves FA, editor. Fundamentos de Limnologia. Rio de Janeiro: Interciência; 2011.
- Duarte CM. Use of echosounder tracings to estimate the aboveground biomass of submerged plants in lakes. Can J Fish Aquat Sci. 1987;44(4):732-735. doi: 10.1139/f87-088.
- Duarte CM, Kalff J, Peters RH. Patterns in biomass and cover of aquatic macrophytes in Lakes: a test with Florida Lakes. Can J Fish Aquat Sci. 1988;45(11):1976-1982. doi: 10.1139/f86-235.
- Duarte CM, Kalff J. Biomass density and the relationship between submerged macrophyte biomass and plant growth form. Hydrobiologia. 1990;196(1):17-23. doi: 10.1007/BF00008889.
- Velini ED, Galo MLBT, Carvalho FT, Martins D, Cavenaghi AL, Trindade MLB, Bravin LFN, Negrisoli E, Antuniassi UR, Simionato JLA, Santos CAS. Assessment of aquatic plants in the reservoirs of AES-Tietê and development of an integrated control model for the most important species. J Environ Sci Heal. 2005;B40(1):85-101. doi: 10.1081/PFC-200034242.
- Thomaz SM, Pagioro TA, Bini LM, Murphy KJ. Effect of reservoir drawdown on biomass of three species of aquatic macrophytes in a large sub-tropical reservoir (Itaipu, Brazil). Hydrobiologia. 2006;570:53-59. doi: 10.1007/978-1-4020-5390-0_8.
- Pierini SA, Thomaz SM. Effects of limnological and morphometric factors upon Zmin, Zmax and width of Egeria spp stands in a tropical reservoir. Braz Arch Biol Technol. 2009;52(2):387-396. doi: 10.1590/S1516-89132009000200016.
- Bianchini I. Modelos de crescimento e decomposição de macrófitas aquáticas. [Growth and decomposition models of aquatic macrophytes] In: Thomaz SM, Bini M, editors. Ecologia e manejo de macrófitas aquáticas. Maringá: EDUEM; 2003. p.85-126.
- Galo MLBT, Velini ED, Trindade MLB, Santos SCA. Remote satellite sensing to monitor macrophyte dispersion in the Tietê river reservoirs. Planta Daninha. 2002;20:7-20. doi: 10.1590/S0100-83582002000400002.
- Intergraph Corporation. Intergraph Microstation PC, Version 4 User’s Guide. Bentley Systems, Inc. and Intergraph Corporation; 1991. p.333.
- Silva TSF, Costa MPF, Melack JM, Novo E. Remote sensing of aquatic vegetation: Theory and applications. Environ Monit Assess. 2008;140(1):131-145. doi: 10.1007/s10661-007-9855-3.
- Silva TSF, Costa MPF, Melack JM. Spatial and temporal variability of macrophyte cover and productivity in the eastern Amazon floodplain: A remote sensing approach. Remote Sens Environ. 2010;114(9):1998-2010. doi: 10.1016/j.rse.2010.04.007.
- Montefalcone M, Rovere A, Parravicini V, Albertelli G, Morri C, Bianchi CN. Evaluating change in seagrass meadows: A time-framed comparison of Side Scan Sonar maps. Aquat Bot. 2011;104:204-212. doi: 10.1016/j.aquabot.2011.05.009.
- Fritz C, Dörnhöfer K, Schneider T, Geist J, Oppelt N. Mapping submerged aquatic vegetation using rapideye satellite data: The example of Lake Kummerow (Germany). Water. 2017;9:510. doi: 10.3390/w9070510.
- Yadav S, Yoneda M, Susaki J, Tamura M, Ishikawa K, Yamashiki Y. A satellite-based assessment of the distribution and biomass of submerged aquatic vegetation in the optically shallow basin of Lake Biwa. Remote Sens. 2017;9:966. doi: 10.3390/rs9090966.
- Leriche A, Pasqualini V, Boudouresque CF, Bernard G, Bonhomme P, Clabaut P, Denis J. Spatial, temporal and structural variations of a Posidonia oceanica seagrass meadow facing human activities. Aquat Bot. 2006;84(4):287-293. doi: 10.1016/j.aquabot.2005.10.001.
- Sabol BM, Kannenberg J, Skogerboe JG. Integrating acoustic mapping into operational aquatic plant management: A case study in Wisconsin. J Aquat Plant Manage. 2009;47:44-52.
- Rotta LHS, Mishra DR, Watanabe FSW, Rodrigues TWP, Alcantara EH, Imai NN. Analyzing the feasibility of a space-borne sensor (SPOT-6) to estimate the height of Submerged Aquatic Vegetation (SAV) in inland waters. ISPRS J. Photogramm. Remote Sens. 2018;44:341-356. doi: 10.1016/j.isprsjprs.2018.07.011.
- Sabol BM. Innovative techniques for improved hydroacoustic bottom tracking in dense aquatic vegetation. Technical Report. Washington DC: Environmental Laboratory of U.S. Army Engineer Research and Development Center. 2001.
- Valley RD, Drake MT, Anderson CS. Evaluation of alternative interpolation techniques for the mapping of remotely-sensed submersed vegetation abundance. Aquat Bot. 2005;81(1):13-25. doi: 10.1016/j.aquabot.2004.09.002.
- Chamberlain RH, Doering PH, Orlando B, Sabol BM. Comparison of manual and hydroacoustic measurement of seagrass distribution in the Caloosahatchee estuary, Florida. Florida Scientist. 2009.
- Valley RD, Drake MT. Accuracy and precision of hydroacoustic estimates of aquatic vegetation and the repeatability of whole-lake surveys: field tests with a commercial echosounder. Technical Report. St. Paul, MN: Minnesota; Department of Natural Resources. 2005.
- Sabol BM, Melton RE, Chamberlain R, Doering P, Haunert K. Evaluation of a digital echo sounder system for detection of submersed aquatic vegetation. Estuaries Coasts. 2002;25(1):133-141. doi: 10.1007/BF02696057.
- Zhu B, Fitzgerald DG, Hoskins SB, Rudstam LG, Mayer CM, Mills EL. Quantification of historical changes of submerged aquatic vegetation cover in two bays of Lake Ontario with three complementary methods. J Great Lakes Res. 2007;33(1):122-135. doi: 10.3394/0380-1330(2007)33[122:QOHCOS]2.0.CO;2.
- Biosonics. User Guide: EcoSAV 1; Biosonics Inc: Seattle, WD, USA; 2008. p.1-48.
- Câmara G, Souza RCM, Freitas UM, Garrido J. SPRING: Integrating remote sensingand GIS by object-oriented data modelling. Comput. Gr. 1996;20(3):395-403. doi: 10.1016/0097-8493(96)00008-8.
- Soares-Filho B, Cerqueira G, Pennachin C. DINAMICA-A stochastic cellular automata model designed to simulate the landscape dynamics in an Amazonian colonization frontier. Ecol model 2002;154:217-235. doi: 10.1016/S0304-3800(02)00059-5.
- Ziaii M, Carranza EJM, Ziaei M. Application of geochemical zonality coefficients in mineral prospectivity mapping. Comput. Geosci. 2011;37(12):1935-1945. doi: 10.1016/j.cageo.2011.05.009.
- Thapa RB, Murayama Y. Urban growth modeling of Kathmandu metropolitan region, Nepal. Comput Environ Urban Syst. 2011;35(1):25-34. doi: 10.1016/j.compenvurbsys.2010.07.005.
- Romero-Calcerrada R, Barrio-Parra F, Millington JDA, Novillo CJ. Spatial modelling of socioeconomic data to understand patterns of human-caused wildfire ignition risk in the SW of Madrid (central Spain). Ecol Model. 2010;221(1):34-45. doi: 10.1016/j.ecolmodel.2009.08.008.
- Fan D, Cui X, Yuan D, Wang J, Yang J, Wang S. Weight of evidence method and its applications and development. 2011. 2nd International Conference on Challenges in Environmental Science and Computer Engineering (CESCE 2011), Procedia Environmental Sciences 11, Part C. 2011;1412-1418.
- Bonham-Carter GF. Geographic Information Systems for Geoscientists: Modelling with GIS. Vol. 13. Computer Methods in the Geosciences. Ontario: Pergamon; 1994.
- Soares-Filho BS. Rodrigues H, Costa W. Modeling Environmental Dynamics with Dinamica EGO. Belo Horizonte: Remote Sensing Center. Federal University of Minas Gerais. 2009.
- Intergraph Corporation. Intergraph Microstation PC, Version 4 User’s Guide. Bentley Systems, Inc. and Intergraph Corporation; 1991. p.333.
- Piñeiro G, Perelman S, Guerschman JP, Paruelo JM. How to evaluate models: Observed vs. predicted or predicted vs. observed? Ecol Model. 2008;216(3-4):316-322. doi: 10.1016/j.ecolmodel.2008.05.006.
- Carrillo Y, Guarín A, Guillot G. Biomass distribution, growth and decay of Egeria densa in a tropical high-mountain reservoir (NEUSA, Colombia). Aquat Bot. 2006;85:7-15. doi: 10.1016/j.aquabot.2006.01.006.
- Duarte CM, Kalff J. Littoral Slope as a predictor of the maximum biomass of submerged macrophyte communities. Limnol Oceanogr. 1986;31(5):1072-1080. doi: 10.4319/lo.1986.31.5.1072.
- Cook CDK, Urmi-König K. A revision of the genus Egeria (Hydrocharitaceae). Aquat Bot. 1984;19:73-96. doi: 10.1016/0304-3770(84)90009-3.
- Rotta LHS, Mishra DR, Alcantara E, Imai NN, Watanabe FSW, Rodrigues T. Kd(PAR) and a depth based model to estimate the height of submerged aquatic vegetation in an oligotrophic reservoir: A case study at Nova Avanhandava. Remote Sens. 2019;11(3):317. doi: 10.3390/rs11030317.
- Bini, LM, Thomaz SM. Prediction of Egeria najas and Egeria densa ocurrence in a large subtropical reservoir (Itaipu Reservoir, Brazil-Paraguay). Aquat Bot. 2005;83:227-238. doi: 10.1016/j.aquabot.2005.06.010.
- Bravin LFN, Velini ED, Reigotta C, Negrisoli E, Corrêa MR, Carbonari CA. Development of equipment for mechanical control of aquatic plants at the Americana reservoir in Brazil. Planta Daninha (Viçosa-MG). 2005;23(2):263-267.
- Nascimento PRF, Pereira SMB, Sampaio EVSB. Egeria densa biomass in the Paulo Afonso-Bahia hydroelectric plant. Planta Daninha. 2008;26(3):481-486. doi: 10.1590/S0100-83582008000300002.
- Sousa WTZ, Thomaz SM, Murphy KJ. Response of native Egeria najas Planch and invasive Hydrilla verticillata (L.f.) Royle to altered hydroecological regime in a subtropical river. Aquat Bot. 2010;92(1):40-48. doi: 10.1016/j.aquabot.2009.10.002.
- Rodrigues RR, Thomaz SM. Photosynthetic and growth responses of Egeria densa to photosynthetic active radiation. Aquat Bot. 2010;92(4):281-284. doi: 10.1016/j.aquabot.2010.01.009.
- Feitosa MF. Comparative analysis of diet, feeding selectivity and structure of the ichthyofauna, juveniles and small fish, in lateral lagoons of Rosana Reservoir (Paranapanema River, SP/PR). [dissertation]. Instituto de Biociências da Universidade Estadual Paulista: UNESP. 2011.