Clement G Yedjou*, Solange S Tchounwou, Jameka Grigsby, Keara Johnson and Paul B Tchounwou
Volume3-Issue8
Dates: Received: 2022-08-23 | Accepted: 2022-08-28 | Published: 2022-08-30
Pages: 980-984
Abstract
Breast Cancer (BC) is the most common malignancy in women worldwide. In the United States, the lifetime risk of developing an invasive form of breast cancer is 12.5% among women. BC arises in the lining cells (epithelium) of the ducts or lobules in the glandular tissue of the breast. The goal of the present study was to use Machine Learning (ML) as a novel technology to assess and compare the invasive forms of BC including, infiltrating ductal carcinoma, infiltrating lobular carcinoma, and mucinous carcinoma. To achieve this goal, we used ML algorithms and collected a dataset of 334 BC patients available at https://www.kaggle.com/amandam1/breastcancerdataset and interpreted this dataset based on the form of BC, age, sex, tumor stages, surgery type, and survival rate. Among the 334 patients, 70% were diagnosed with infiltrating ductal carcinoma, 27% with infiltrating lobular carcinoma, and 3% with mucinous carcinoma. Overall, out of 334 BC patients: 64 (19.16%) were in stage I, 189 (56.59%) in stage II, and 81 (24.25%) in stage III. Sixty-six, 67, 96, and 105 patients underwent lumpectomy, simple mastectomy, modified radical mastectomy, and other types of surgery, respectively. The survival rates were 83.4% for stage I, 79.1% for stage II, and 77% for stage III. Findings from the present study demonstrated that ML provides an important tool to curate large amount of BC data, as well as a scientific means to improve BC outcomes.
FullText HTML
FullText PDF
DOI: 10.37871/jbres1540
Certificate of Publication
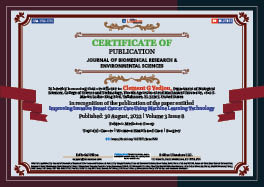
Copyright
© 2022 Yedjou CG, et al. Distributed under Creative Commons CC-BY 4.0
How to cite this article
Yedjou CG, Tchounwou SS, Grigsby J, Johnson K, Tchounwou PB. Improving Invasive Breast Cancer Care Using Machine Learning Technology. J Biomed Res Environ Sci. 2022 Aug 30; 3(8): 980-984. doi: 10.37871/jbres1540, Article ID: JBRES1540, Available at: https://www.jelsciences.com/articles/jbres1540.pdf
Subject area(s)
References
- Ferlay J, Colombet M, Soerjomataram I, Parkin DM, Piñeros M, Znaor A, Bray F. Cancer statistics for the year 2020: An overview. Int J Cancer. 2021 Apr 5. doi: 10.1002/ijc.33588. Epub ahead of print. PMID: 33818764.
- Bray F, Ferlay J, Soerjomataram I, Siegel RL, Torre LA, Jemal A. Global cancer statistics 2018: GLOBOCAN estimates of incidence and mortality worldwide for 36 cancers in 185 countries. CA Cancer J Clin. 2018 Nov;68(6):394-424. doi: 10.3322/caac.21492. Epub 2018 Sep 12. Erratum in: CA Cancer J Clin. 2020 Jul;70(4):313. PMID: 30207593.
- Siegel RL, Miller KD, Jemal A. Cancer statistics, 2019. CA Cancer J Clin. 2019 Jan;69(1):7-34. doi: 10.3322/caac.21551. Epub 2019 Jan 8. PMID: 30620402.
- DeSantis CE, et al. Breast cancer statistics 2019 CA. Cancer J Clin. 2019;69.
- Hoda SA, Hoda RS. Robbins and Cotran Pathologic Basis of Disease. Am J Clin Pathol. 2020;154.
- Kumar V, Abbas A, Aster J, Turner J. Robbins Cotran Pathologic Basis of Disease Tenth Edition. Elsevier. 2021.
- Badowska-Kozakiewicz AM, Liszcz A, Sobol M, Patera J. Retrospective evaluation of histopathological examinations in invasive ductal breast cancer of no special type: an analysis of 691 patients. Arch Med Sci. 2017 Oct;13(6):1408-1415. doi: 10.5114/aoms.2015.53964. Epub 2016 Mar 4. PMID: 29181072; PMCID: PMC5701672.
- Chand P, Garg A, Singla V, Rani N. Evaluation of Immunohistochemical Profile of Breast Cancer for Prognostics and Therapeutic Use. Niger J Surg. 2018 Jul-Dec;24(2):100-106. doi: 10.4103/njs.NJS_2_18. PMID: 30283220; PMCID: PMC6158994.
- Tsagkaraki IM, Kourouniotis CD, Gomatou GL, Syrigos NK, Kotteas EA. Orbital metastases of invasive lobular breast carcinoma. Breast Dis. 2019;38(3-4):85-91. doi: 10.3233/BD-190398. PMID: 31640079.
- Suchman K, Zimmerman BS, Ru M, Cascetta KP, Tiersten A. Distribution of oncotype recurrence scores in invasive lobular carcinomas. J Clin Oncol. 2020;38.
- Arpino G, Bardou VJ, Clark GM, Elledge RM. Infiltrating lobular carcinoma of the breast: tumor characteristics and clinical outcome. Breast Cancer Res. 2004;6(3):R149-56. doi: 10.1186/bcr767. Epub 2004 Feb 17. PMID: 15084238; PMCID: PMC400666.
- Cao AY, Huang L, Wu J, Lu JS, Liu GY, Shen ZZ, Shao ZM, Di GH. Tumor characteristics and the clinical outcome of invasive lobular carcinoma compared to infiltrating ductal carcinoma in a Chinese population. World J Surg Oncol. 2012 Jul 17;10:152. doi: 10.1186/1477-7819-10-152. PMID: 22805492; PMCID: PMC3502188.
- Han B, Gu Z, Liu Z, Ling H. Clinical Characteristics and Survival Outcomes of Infiltrating Lobular Carcinoma: A Retrospective Study of 365 Cases in China. Cancer Manag Res. 2022 Feb 16;14:647-658. doi: 10.2147/CMAR.S346319. PMID: 35210861; PMCID: PMC8858761.
- Johnson K, Sarma D, Hwang ES. Lobular breast cancer series: imaging. Breast Cancer Res. 2015 Jul 11;17(1):94. doi: 10.1186/s13058-015-0605-0. PMID: 26163296; PMCID: PMC4499185.
- Shakoor MT, Ayub S, Mohindra R, Ayub Z, Ahad A. Unique presentations of invasive lobular breast cancer: a case series. Int J Biomed Sci. 2014 Dec;10(4):287-93. PMID: 25598762; PMCID: PMC4289705.
- Li CI, Uribe DJ, Daling JR. Clinical characteristics of different histologic types of breast cancer. Br J Cancer. 2005 Oct 31;93(9):1046-52. doi: 10.1038/sj.bjc.6602787. PMID: 16175185; PMCID: PMC2361680.
- Zhao S, Ma D, Xiao Y, Jiang YZ, Shao ZM. Clinicopathologic features and prognoses of different histologic types of triple-negative breast cancer: A large population-based analysis. Eur J Surg Oncol. 2018 Apr;44(4):420-428. doi: 10.1016/j.ejso.2017.11.027. Epub 2018 Jan 9. PMID: 29429597.
- Ide Y, Horii R, Osako T, Ogura K, Yoshida R, Iwase T, Akiyama F. Clinicopathological significance of invasive micropapillary carcinoma component in invasive breast carcinoma. Pathol Int. 2011 Dec;61(12):731-6. doi: 10.1111/j.1440-1827.2011.02735.x. Epub 2011 Oct 31. PMID: 22126380.
- Fisher ER, Dignam J, Tan-Chiu E, Costantino J, Fisher B, Paik S, Wolmark N. Pathologic findings from the National Surgical Adjuvant Breast Project (NSABP) eight-year update of Protocol B-17: intraductal carcinoma. Cancer. 1999 Aug 1;86(3):429-38. doi: 10.1002/(sici)1097-0142(19990801)86:3<429::aid-cncr11>3.0.co;2-y. PMID: 10430251.
- Gokce H, Durak MG, Akin MM, Canda T, Balci P, Ellidokuz H, Demirkan B, Gorken IB, Sevinc AI, Kocdor MA, Saydam S, Harmancioglu O. Invasive micropapillary carcinoma of the breast: a clinicopathologic study of 103 cases of an unusual and highly aggressive variant of breast carcinoma. Breast J. 2013 Jul-Aug;19(4):374-81. doi: 10.1111/tbj.12128. Epub 2013 May 29. PMID: 23714006.
- Kaoku S, Konishi E, Fujimoto Y, Tohno E, Shiina T, Kondo K, Yamazaki S, Kajihara M, Shinkura N, Yanagisawa A. Sonographic and pathologic image analysis of pure mucinous carcinoma of the breast. Ultrasound Med Biol. 2013 Jul;39(7):1158-67. doi: 10.1016/j.ultrasmedbio.2013.02.014. Epub 2013 May 15. PMID: 23683410.
- Xu X, Bi R, Shui R, Yu B, Cheng Y, Tu X, Yang W. Micropapillary pattern in pure mucinous carcinoma of the breast - does it matter or not? Histopathology. 2019 Jan;74(2):248-255. doi: 10.1111/his.13722. Epub 2018 Oct 23. PMID: 30066338.
- Zhang H, Qiu L, Peng Y. The sonographic findings of micropapillary pattern in pure mucinous carcinoma of the breast. World J Surg Oncol. 2018 Jul 24;16(1):151. doi: 10.1186/s12957-018-1449-8. PMID: 30041628; PMCID: PMC6058370.
- Kesson EM, Allardice GM, George WD, Burns HJ, Morrison DS. Effects of multidisciplinary team working on breast cancer survival: retrospective, comparative, interventional cohort study of 13 722 women. BMJ. 2012 Apr 26;344:e2718. doi: 10.1136/bmj.e2718. PMID: 22539013; PMCID: PMC3339875.
- Sharma G, Prabha C. Applications of Machine Learning in Cancer Prediction and Prognosis. in Cancer Prediction for Industrial IoT 4.0: A Machine Learning Perspective. 2021. doi:10.1201/9781003185604-8
- Deepti, Ray SA. Survey on Application of Machine Learning Algorithms in Cancer Prediction and Prognosis. In Advances in Intelligent Systems and Computing. 2021;1174.
- Yedjou CG, Tchounwou SS, Aló RA, Elhag R, Mochona B, Latinwo L. Application of Machine Learning Algorithms in Breast Cancer Diagnosis and Classification. Int J Sci Acad Res. 2021 Jan;2(1):3081-3086. Epub 2021 Oct 30. PMID: 34825131; PMCID: PMC8612371.
- Mosquera-Lopez C, Agaian S, Velez-Hoyos A, Thompson I. Computer-Aided Prostate Cancer Diagnosis From Digitized Histopathology: A Review on Texture-Based Systems. IEEE Rev Biomed Eng. 2015;8:98-113. doi: 10.1109/RBME.2014.2340401. Epub 2014 Jul 17. PMID: 25055385.
- Nir G, Karimi D, Goldenberg SL, Fazli L, Skinnider BF, Tavassoli P, Turbin D, Villamil CF, Wang G, Thompson DJS, Black PC, Salcudean SE. Comparison of Artificial Intelligence Techniques to Evaluate Performance of a Classifier for Automatic Grading of Prostate Cancer From Digitized Histopathologic Images. JAMA Netw Open. 2019 Mar 1;2(3):e190442. doi: 10.1001/jamanetworkopen.2019.0442. PMID: 30848813; PMCID: PMC6484626.
- Shishido SN, Faulkner EB, Beck A, Nguyen TA. The effect of antineoplastic drugs in a male spontaneous mammary tumor model. PLoS One. 2013 Jun 3;8(6):e64866. doi: 10.1371/journal.pone.0064866. PMID: 23755153; PMCID: PMC3670867.
- Ellington TD, Henley SJ, Wilson RJ, Miller JW. Breast Cancer Survival Among Males by Race, Ethnicity, Age, Geographic Region, and Stage - United States, 2007-2016. MMWR Morb Mortal Wkly Rep. 2020 Oct 16;69(41):1481-1484. doi: 10.15585/mmwr.mm6941a2. PMID: 33056954; PMCID: PMC7561088.
- Flaherty DC, Bawa R, Burton C, Goldfarb M. Breast Cancer in Male Adolescents and Young Adults. Ann Surg Oncol. 2017 Jan;24(1):84-90. doi: 10.1245/s10434-016-5586-4. Epub 2016 Sep 20. PMID: 27650826.
- McPherson K, Steel CM, Dixon JM. ABC of breast diseases. Breast cancer-epidemiology, risk factors, and genetics. BMJ. 2000 Sep 9;321(7261):624-8. doi: 10.1136/bmj.321.7261.624. PMID: 10977847; PMCID: PMC1118507.
- Frank S, Carton M, Dubot C, Campone M, Pistilli B, Dalenc F, Mailliez A, Levy C, D'Hondt V, Debled M, Vermeulin T, Coudert B, Perrin C, Gonçalves A, Uwer L, Ferrero JM, Eymard JC, Petit T, Mouret-Reynier MA, Patsouris A, Guesmia T, Bachelot T, Robain M, Cottu P. Impact of age at diagnosis of metastatic breast cancer on overall survival in the real-life ESME metastatic breast cancer cohort. Breast. 2020 Aug;52:50-57. doi: 10.1016/j.breast.2020.04.009. Epub 2020 Apr 23. PMID: 32380440; PMCID: PMC7375638.
- Lofterød T, Frydenberg H, Flote V, Eggen AE, McTiernan A, Mortensen ES, Akslen LA, Reitan JB, Wilsgaard T, Thune I. Exploring the effects of lifestyle on breast cancer risk, age at diagnosis, and survival: the EBBA-Life study. Breast Cancer Res Treat. 2020 Jul;182(1):215-227. doi: 10.1007/s10549-020-05679-2. Epub 2020 May 20. PMID: 32436147; PMCID: PMC7275030.
- Brandt J, Garne JP, Tengrup I, Manjer J. Age at diagnosis in relation to survival following breast cancer: a cohort study. World J Surg Oncol. 2015 Feb 7;13:33. doi: 10.1186/s12957-014-0429-x. PMID: 25889186; PMCID: PMC4344734.
- Aebi S, Castiglione M. The enigma of young age. Ann Oncol. 2006 Oct;17(10):1475-7. doi: 10.1093/annonc/mdl330. PMID: 17005630.
- Noone AM, et al. (eds). Cancer Statistics Review, 1975-2015 - SEER Statistics. SEER Cancer Statistics Review. 2017.
- Berry DA, Cronin KA, Plevritis SK, Fryback DG, Clarke L, Zelen M, Mandelblatt JS, Yakovlev AY, Habbema JD, Feuer EJ; Cancer Intervention and Surveillance Modeling Network (CISNET) Collaborators. Effect of screening and adjuvant therapy on mortality from breast cancer. N Engl J Med. 2005 Oct 27;353(17):1784-92. doi: 10.1056/NEJMoa050518. PMID: 16251534.
- Munoz D, Near AM, van Ravesteyn NT, Lee SJ, Schechter CB, Alagoz O, Berry DA, Burnside ES, Chang Y, Chisholm G, de Koning HJ, Ali Ergun M, Heijnsdijk EA, Huang H, Stout NK, Sprague BL, Trentham-Dietz A, Mandelblatt JS, Plevritis SK. Effects of screening and systemic adjuvant therapy on ER-specific US breast cancer mortality. J Natl Cancer Inst. 2014 Sep 24;106(11):dju289. doi: 10.1093/jnci/dju289. PMID: 25255803; PMCID: PMC4271026.
- Luo T, Spolverato G, Johnston F, Haider AH, Pawlik TM. Factors that determine cancer treatment choice among minority groups. J Oncol Pract. 2015 May;11(3):259-61. doi: 10.1200/JOP.2015.003640. Epub 2015 Mar 3. PMID: 25736381.