Jin Wang, Youjun Jiang, Li Li, Chao Yang, Ke Li, Xueping Lan, Yuchong Zhang and Jinying Chen*
Volume2-Issue8
Dates: Received: 2021-08-12 | Accepted: 2021-08-17 | Published: 2021-08-18
Pages: 675-684
Abstract
The purpose of grain storage management is to dynamically analyze the quality change of the reserved grains, adopt scientific and effective management methods to delay the speed of the quality deterioration, and reduce the loss rate during storage. At present, the supervision of the grain quality in the reserve mainly depends on the periodic measurements of the quality of the grains and the milled products. The data obtained by the above approach is accurate and reliable, but the workload is too large while the frequency is high. The obtained conclusions are also limited to the studied area and not applicable to be extended into other scenarios. Therefore, there is an urgent need of a general method that can quickly predict the quality of grains given different species, regions and storage periods based on historical data. In this study, we introduced Back-Propagation (BP) neural network algorithm and support vector machine algorithm into the quality prediction of the reserved grains. We used quality index, temperature and humidity data to build both an intertemporal prediction model and a synchronous prediction model. The results show that the BP neural network based on the storage characters from the first three periods can accurately predict the key storage characters intertemporally. The support vector machine can provide precise predictions of the key storage characters synchronously. The average predictive error for each of wheat, rice and corn is less than 15%, while the one for soybean is about 20%, all of which can meet the practical demands. In conclusion, the machine learning algorithms are helpful to improve the management effectiveness of grain storage.
FullText HTML
FullText PDF
DOI: 10.37871/jbres1296
Certificate of Publication
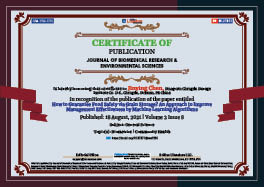
Copyright
© 2021 Wang J, et al. Distributed under Creative Commons CC-BY 4.0
How to cite this article
Wang J, Jiang Y, Li L, Yang C, Li K, Lan X, Zhang Y, Chen J. How to Guarantee Food Safety via Grain Storage? An Approach to Improve Management Effectiveness by Machine Learning Algorithms. J Biomed Res Environ Sci. 2021 Aug 18; 2(8): 675-684. doi: 10.37871/jbres1296, Article ID: JBRES1296, Available at: https://www.jelsciences.com/articles/jbres1296.pdf
Subject area(s)
University/Institute
References
- Cheng Du. Study on the process of different grain storage ecological regions in China. Sichuan science and technology press. 2014.
- Hui L, Jianxin Z, Yong F, Yulong G, Weifen Q. Microorganism and quality changes during paddy storage. Journal of the Chinese Cereals and Oils Association. 2020;35:126-131.
- Wang RL, Li DL, Tian ZQ, Kong XG, Dong GY, Su K. Change rule of maize quality under controlled atmosphere storage. Journal of Henan University of Technology. 2011;32:1-5
- Chao ZMCKG. Quality change of early indica rice storage in guangdong. Grain Science and Technology and Economy. 2016;41:57-59
- Wei Z, Wang J, Li X, Li L. Study on Quality Changes of Harvested Wheat in Storage Process. Academic Periodical of Farm Products Processing. 2009;92-95
- Zhan Q. The development history and application prospect of machine learning. Public Communication of Science & Technology. 2018;10:138-139
- Hu L, Liu T, Li H, Cui y. Prospects for machine learning research and its application in agriculture. Agricultural Library and Information. 2019;31:12-22. https://tinyurl.com/43k3bhfr
- Ma D, Zhou T, Chen J, Qi S, Shahzad M, Xiao Z. Supercritical water heat transfer coefficient prediction analysis based on BP neural network. Nuclear Engineering and Design. 2017;320:400-408. https://tinyurl.com/2dyjd8ws
- Xu B, Dan HC, Li L. Temperature prediction model of asphalt pavement in cold regions based on an improved BP neural network. Applied Thermal Engineering. 2017;120:568-580. https://tinyurl.com/9zywxz4
- Yu S. Recognition of locomotion patterns based on BP neural network during different walking speeds. 2017 Chinese Automation Congress. 2017. https://tinyurl.com/43h6v7dc
- Tu W, Zhong S, Shen Y, Incecik A, Fu X. Neural network-based hybrid signal processing approach for resolving thin marine protective coating by terahertz pulsed imaging. Ocean Engineering. 2019;173:58-67. https://tinyurl.com/t6s63rue
- Jiang N, Gu Q, Yang H, Huang J. Machine learning algorithm under big date. Computer and Information Technology. 2019;27:30-33
- Mao Z, Sun Y. Study on a recognition algorithm of corn tassel image. Journal of Anhui Agricultural Sciences. 2018;46:193-195.
- Liu T, Wang T, Hu L. Rhizocotonia Solani Recognition algorithm based on convolutional neural network. Chinese Journal of Rice Science. 2019;33:90-94
- Gu Z, Zhang Z, Sun J, Li B. Robust image recognition by L1-norm twin-projection support vector machine. Neurocomputing. 2017;223:1-11. https://tinyurl.com/nsewzk7d
- Wasan S, Ahmed A, Ahmed A. Face Recognition Approach using an Enhanced Particle Swarm Optimization and Support Vector Machine. Journal of Engineering and Applied Sciences. 2019;14:2982-2987. https://tinyurl.com/846cbxzu
- Chandaka S, Chatterjee A, Munshi S. Support vector machines employing cross-correlation for emotional speech recognition. Measurement. 2009;42:611-618. https://tinyurl.com/5cht4rwb
- Vishal Naik, Online Handwritten Gujarati Numeral Recognition Using Support Vector Machine. International Journal of Computer Sciences and Engineering. 2018;6:416-420. https://tinyurl.com/4dy2txvx
- Lam L, Rajprasad R, Dino I. An enhanced Support Vector Machine classification framework by using Euclidean distance function for text document categorization. Applied Intelligence. 2011;37:80-99. https://tinyurl.com/yf7x5dms
- Noble WS. What is a support vector machine? Nature Biotechnology. 2006;24:1565-1567. https://tinyurl.com/hjruct7t
- Tripathy. Rice crop yield prediction in India using support vector machines in 2016. 13th International Joint Conference on Computer Science and Software Engineering (JCSSE). 2016. https://tinyurl.com/466xhr48
- Yi D, Wei C, Ping Z, Shu Z. Regression method based on SVM classification and its application in production forecast. Journal of Computer Applications. 2010;30:2310-2313. https://tinyurl.com/6h6srmxv
- Huang T, Yang R, Huang W, Huang Y, Qiao X. Detecting sugarcane borer diseases using support vector machine. Information Processing in Agriculture. 2018;5(1):74-82 https://tinyurl.com/x79jexs
- Singh K, Kumar S, Kaur P. Support vector machine classifier based detection of fungal rust disease in Pea Plant (Pisam sativam). International Journal of Information Technology. 2019;11:485-492. https://tinyurl.com/bs7cjya9
- Shang ZZ, Weimin. The application of data mining in grain storage. Microcomputer Information. 2006;22:192-194.
- Gnana K, Deepa SN. Neural network based hybrid computing model for wind speed prediction. Neurocomputing. 2013;122:425-429. https://tinyurl.com/2dvkyc89
- Ma CM, Yang WS, Cheng BW. How the Parameters of K-nearest Neighbor Algorithm Impact on the Best Classification Accuracy: In Case of Parkinson Dataset. Journal of Applied Sciences. 2014;14:171-176. https://tinyurl.com/38h4nwm2
- Wang X, Liu T, Zheng X, Peng H, Xin J, Zhang B. Short-term prediction of groundwater level using improved random forest regression with a combination of random features. Applied Water Science. 2018;8:125. https://tinyurl.com/3bvsjeaj
- Heydari M, Olyaie E, Mohebzadeh H, Kisi O. Development of a Neural Network Technique for Prediction of Water Quality Parameters in the Delaware River, Pennsylvania. 2013. https://tinyurl.com/5fr548jh